In an age where artificial intelligence (AI) drives everything from your social media feed to loan approvals, one uncomfortable truth stands out: AI is not always fair. This phenomenon, known as AI bias, is becoming one of the most hotly debated issues in the tech world and beyond. But what exactly is AI bias? How does it emerge? And why should we care?
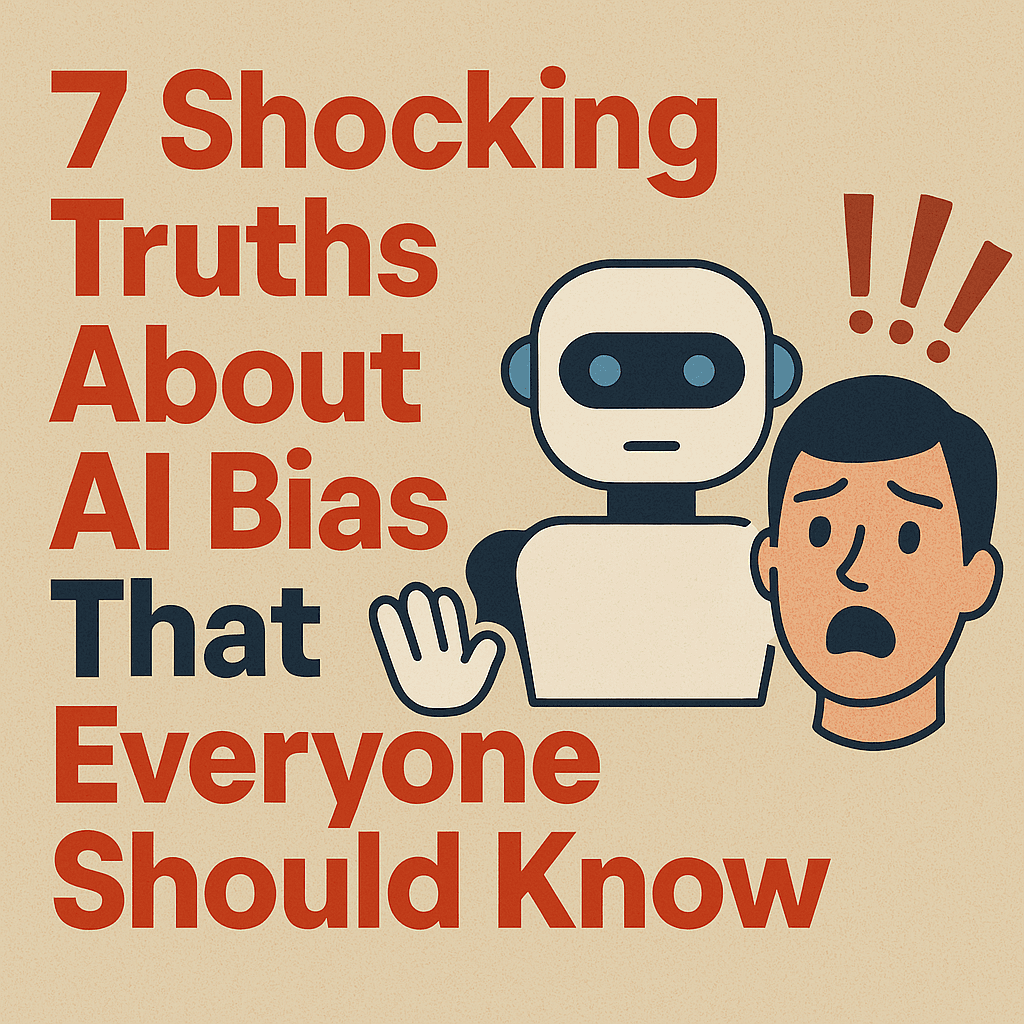
In this in-depth guide, we break down AI bias, how it impacts individuals and society, and what can be done to minimize its effects.
What is AI Bias?
AI bias refers to systematic and unfair discrimination in the outcomes produced by AI algorithms. This bias can manifest in various ways, such as favoring one gender, ethnicity, or socioeconomic group over another.
In essence, AI bias occurs when an algorithm produces results that are prejudiced due to erroneous assumptions in the machine learning process. These biases are not always intentional, but their consequences can be far-reaching and damaging.
What Are Some Recent Examples of AI Bias?
- Apple Card Gender Discrimination (2019): Apple was criticized after users reported that women were offered lower credit limits than men despite having similar financial profiles. This sparked a public outcry and an investigation by regulators.
- COMPAS Criminal Justice System (Ongoing): The COMPAS algorithm used in U.S. courts to assess the risk of recidivism has been shown to be biased against Black defendants, often rating them as higher risk than white defendants charged with similar crimes.
- Google Photos (2023): Google’s image recognition tool mistakenly tagged images of Black individuals with inappropriate labels due to flawed training data. Google had to pull the feature and commit to retraining the model.
- AI in Education (2021–2024): During the COVID-19 pandemic, the UK used an algorithm to predict student grades based on past school performance. The system disproportionately downgraded students from less privileged schools, leading to widespread criticism.
What is Sample Bias in AI?
Sample bias occurs when the data used to train an AI system is not representative of the real-world population it’s intended to serve. This leads to skewed outcomes and poor generalization when the model is deployed.
Examples:
- A facial recognition model trained mainly on images of light-skinned individuals will perform poorly on darker-skinned individuals.
- A language model trained on Western media might not accurately interpret idioms or contexts specific to other cultures.
Sample bias is one of the most common and foundational causes of AI bias.
Also read – 7 Proven Methods To Exit A Non Performing Stock
How Does AI Bias Happen?
AI systems are only as good as the data they are trained on. When that data contains historical biases, the algorithm learns and perpetuates those patterns.
Key Reasons Why AI Bias Occurs:
- Biased Training Data: If the data used to train an AI system is unrepresentative or skewed, the algorithm will inherit those biases. For example, if a facial recognition dataset contains more white faces than non-white ones, the model will perform better on white individuals.
- Historical Inequities: AI often learns from human-generated data, which can reflect past and present societal inequities. Algorithms used for loan approvals may learn from decades of biased lending practices.
- Labeling Errors: Data labeling is often done by humans, who bring their own biases. These can be accidentally transferred into the dataset.
- Imbalanced Objectives: Sometimes, models are optimized for accuracy without considering fairness. This can lead to efficient but inequitable outcomes.
- Feedback Loops: AI systems that make decisions (e.g., predicting crime hotspots) can create self-fulfilling prophecies. If police patrol more in one area due to AI predictions, more crimes are reported there, reinforcing the bias.
What Are 3 Sources of Bias in AI?
- Data Bias: Arises from unbalanced or non-representative training data. This includes sample bias, selection bias, and measurement bias.
- Algorithmic Bias: Happens when the model itself introduces or amplifies biases during processing, often due to flawed assumptions or optimization criteria.
- Human Bias: Enters through decisions made during data collection, labeling, or feature engineering. Developers and data scientists may unintentionally embed their own biases.
Why AI Bias Matters
AI bias is not just a tech issue—it’s a societal issue. The decisions made by biased algorithms can affect job opportunities, healthcare access, legal outcomes, and even freedom.
Key Consequences:
- Discrimination: People can be unfairly treated based on race, gender, or socioeconomic status.
- Loss of Trust: Biased AI can erode public trust in technology and institutions.
- Widening Inequality: Biased systems can exacerbate existing inequalities rather than correct them.
- Legal and Ethical Concerns: Using biased algorithms in critical sectors may violate anti-discrimination laws and ethical standards.
Is AI Bias Inevitable?
The short answer is no—but it requires conscious effort to avoid. While AI bias is a byproduct of human bias, it can be mitigated with the right tools and practices.
How to Stop AI Bias?
- Use Diverse Datasets: Ensure training data includes a wide range of demographics and real-world scenarios.
- Regular Bias Audits: Routinely test AI systems for biased outcomes using fairness metrics.
- Apply Fairness Constraints: Implement algorithmic constraints that promote equal treatment across groups.
- Adopt Ethical Frameworks: Follow principles like FAT (Fairness, Accountability, Transparency).
- Enable Explainability: Develop Explainable AI (XAI) that allows users to understand how decisions are made.
- Engage Multidisciplinary Teams: Involve ethicists, sociologists, and affected communities in AI development.
- Establish Regulations and Oversight: Governments and institutions must create enforceable standards for ethical AI.
The Future of AI and Bias
The conversation around AI bias is gaining momentum globally. Major tech companies like Google, Microsoft, and IBM are investing in fairness research and responsible AI. Governments are beginning to legislate for algorithmic transparency and ethical AI development.
Yet, there is a long way to go.
Trends to Watch:
- Explainable AI (XAI): Making AI decisions more understandable to humans.
- AI Governance Laws: Expect more legal frameworks to emerge globally.
- Bias Detection Tools: New tools are emerging to help detect bias in datasets and models.
- AI Ethics Committees: Companies and institutions are forming boards to oversee responsible AI practices.
Final Thoughts
AI is shaping the future—but it shouldn’t mirror the worst of our past. Understanding AI bias is the first step in demanding better, fairer technology. As users, developers, and citizens, we have the power to question how these systems work and push for ethical standards.
Because if we don’t teach AI to be fair, it will simply learn from us. And history, as we know, hasn’t always been just.
Stay informed. Stay logical.
Visit The Logic Stick for more deep dives and explainers that make sense of the world around you.